Machine Generated Data
Tags
Amazon
created on 2019-06-04
Human | 99.1 | |
| ||
Person | 99.1 | |
| ||
Person | 98.8 | |
| ||
Person | 98.3 | |
| ||
Person | 98.2 | |
| ||
Person | 98.1 | |
| ||
Person | 98 | |
| ||
Person | 97.2 | |
| ||
Person | 97 | |
| ||
Person | 92.9 | |
| ||
Room | 92.7 | |
| ||
Classroom | 92.7 | |
| ||
Indoors | 92.7 | |
| ||
School | 92.7 | |
| ||
Text | 86.6 | |
| ||
Person | 84.1 | |
| ||
Person | 84.1 | |
| ||
Person | 84 | |
| ||
Person | 81.7 | |
| ||
Person | 81.5 | |
| ||
Person | 77.4 | |
| ||
Person | 75.8 | |
| ||
Person | 75.7 | |
| ||
Person | 74.3 | |
| ||
Person | 74.3 | |
| ||
Person | 69.5 | |
| ||
Person | 69.3 | |
| ||
Person | 65.2 | |
| ||
Person | 61.8 | |
| ||
Page | 57.9 | |
| ||
Advertisement | 57 | |
| ||
Person | 52.3 | |
| ||
Person | 48.5 | |
| ||
Person | 46.9 | |
|
Clarifai
created on 2019-06-04
Imagga
created on 2019-06-04
sketch | 100 | |
| ||
drawing | 91.2 | |
| ||
representation | 67.3 | |
| ||
vintage | 31.4 | |
| ||
grunge | 28.1 | |
| ||
old | 27.9 | |
| ||
paper | 23.6 | |
| ||
money | 22.1 | |
| ||
retro | 21.3 | |
| ||
business | 20.7 | |
| ||
antique | 18.4 | |
| ||
architecture | 18 | |
| ||
house | 17.5 | |
| ||
ancient | 17.3 | |
| ||
aged | 17.2 | |
| ||
currency | 17.1 | |
| ||
wheeled vehicle | 16.7 | |
| ||
finance | 16.1 | |
| ||
freight car | 16 | |
| ||
texture | 16 | |
| ||
bill | 15.2 | |
| ||
dollar | 14.9 | |
| ||
symbol | 14.8 | |
| ||
cash | 14.6 | |
| ||
bank | 14.5 | |
| ||
frame | 14.2 | |
| ||
design | 14.1 | |
| ||
car | 13.8 | |
| ||
letter | 13.8 | |
| ||
building | 13.5 | |
| ||
art | 13.3 | |
| ||
blank | 12.9 | |
| ||
office | 12.9 | |
| ||
dirty | 12.7 | |
| ||
history | 12.5 | |
| ||
sign | 12 | |
| ||
structure | 11.8 | |
| ||
financial | 11.6 | |
| ||
stamp | 10.9 | |
| ||
postmark | 10.8 | |
| ||
city | 10.8 | |
| ||
postage | 10.8 | |
| ||
wealth | 10.8 | |
| ||
10.5 | ||
| ||
post | 10.5 | |
| ||
wagon | 10.5 | |
| ||
construction | 10.3 | |
| ||
vehicle | 10.3 | |
| ||
note | 10.1 | |
| ||
envelope | 10.1 | |
| ||
space | 10.1 | |
| ||
silhouette | 9.9 | |
| ||
travel | 9.9 | |
| ||
style | 9.6 | |
| ||
worn | 9.5 | |
| ||
empty | 9.4 | |
| ||
card | 9.4 | |
| ||
paint | 9.1 | |
| ||
graphic | 8.8 | |
| ||
artistic | 8.7 | |
| ||
us | 8.7 | |
| ||
pay | 8.6 | |
| ||
grungy | 8.5 | |
| ||
plan | 8.5 | |
| ||
historical | 8.5 | |
| ||
black | 8.4 | |
| ||
savings | 8.4 | |
| ||
banking | 8.3 | |
| ||
historic | 8.2 | |
| ||
container | 8.2 | |
| ||
message | 8.2 | |
| ||
pattern | 8.2 | |
| ||
border | 8.1 | |
| ||
landmark | 8.1 | |
| ||
text | 7.9 | |
| ||
postal | 7.8 | |
| ||
bills | 7.8 | |
| ||
wall | 7.7 | |
| ||
payment | 7.7 | |
| ||
exchange | 7.6 | |
| ||
old fashioned | 7.6 | |
| ||
street | 7.4 | |
|
Google
created on 2019-06-04
Photograph | 95.9 | |
| ||
Text | 92.4 | |
| ||
Snapshot | 84.1 | |
| ||
Room | 76.5 | |
| ||
Collection | 76.1 | |
| ||
Art | 69.5 | |
| ||
History | 67.6 | |
| ||
Adaptation | 67 | |
| ||
Photography | 62.4 | |
|
Color Analysis
Face analysis
Amazon

AWS Rekognition
Age | 17-27 |
Gender | Female, 50.3% |
Angry | 49.5% |
Sad | 50.3% |
Disgusted | 49.5% |
Calm | 49.5% |
Happy | 49.5% |
Surprised | 49.5% |
Confused | 49.5% |

AWS Rekognition
Age | 16-27 |
Gender | Female, 50.3% |
Disgusted | 49.6% |
Happy | 49.6% |
Confused | 49.5% |
Sad | 50% |
Calm | 49.8% |
Angry | 49.6% |
Surprised | 49.5% |

AWS Rekognition
Age | 23-38 |
Gender | Female, 50.2% |
Disgusted | 49.5% |
Surprised | 49.5% |
Confused | 49.5% |
Sad | 50.4% |
Angry | 49.5% |
Calm | 49.5% |
Happy | 49.5% |

AWS Rekognition
Age | 48-68 |
Gender | Female, 50.2% |
Disgusted | 49.7% |
Happy | 49.5% |
Calm | 49.6% |
Angry | 49.6% |
Confused | 49.5% |
Sad | 50% |
Surprised | 49.6% |

AWS Rekognition
Age | 26-43 |
Gender | Female, 50.2% |
Sad | 49.8% |
Surprised | 49.5% |
Calm | 49.7% |
Happy | 49.7% |
Disgusted | 49.5% |
Confused | 49.6% |
Angry | 49.6% |

AWS Rekognition
Age | 23-38 |
Gender | Male, 50.3% |
Calm | 49.6% |
Disgusted | 49.6% |
Angry | 49.6% |
Sad | 50.2% |
Happy | 49.5% |
Confused | 49.5% |
Surprised | 49.5% |

AWS Rekognition
Age | 23-38 |
Gender | Female, 50.4% |
Confused | 49.6% |
Calm | 49.5% |
Disgusted | 49.6% |
Happy | 49.7% |
Angry | 49.6% |
Sad | 49.7% |
Surprised | 49.6% |

AWS Rekognition
Age | 17-27 |
Gender | Male, 50.5% |
Happy | 49.5% |
Angry | 50.1% |
Confused | 49.5% |
Disgusted | 49.6% |
Sad | 49.8% |
Calm | 49.5% |
Surprised | 49.5% |

AWS Rekognition
Age | 35-53 |
Gender | Female, 50.4% |
Calm | 49.5% |
Disgusted | 49.8% |
Angry | 49.6% |
Sad | 50% |
Happy | 49.6% |
Confused | 49.5% |
Surprised | 49.5% |

AWS Rekognition
Age | 27-44 |
Gender | Female, 50.2% |
Happy | 49.5% |
Confused | 49.8% |
Surprised | 49.6% |
Angry | 49.7% |
Calm | 49.5% |
Sad | 49.9% |
Disgusted | 49.6% |

AWS Rekognition
Age | 29-45 |
Gender | Female, 50.4% |
Happy | 49.5% |
Angry | 49.6% |
Confused | 49.7% |
Calm | 49.8% |
Sad | 49.8% |
Disgusted | 49.5% |
Surprised | 49.6% |

AWS Rekognition
Age | 11-18 |
Gender | Female, 50.5% |
Confused | 49.6% |
Angry | 49.6% |
Happy | 49.6% |
Sad | 49.9% |
Disgusted | 49.6% |
Surprised | 49.6% |
Calm | 49.8% |

AWS Rekognition
Age | 16-27 |
Gender | Male, 50.5% |
Surprised | 49.6% |
Angry | 49.6% |
Calm | 49.5% |
Happy | 49.5% |
Sad | 49.9% |
Disgusted | 49.5% |
Confused | 49.8% |

AWS Rekognition
Age | 26-43 |
Gender | Female, 50.3% |
Angry | 49.6% |
Sad | 50% |
Surprised | 49.5% |
Confused | 49.6% |
Disgusted | 49.5% |
Happy | 49.6% |
Calm | 49.6% |

AWS Rekognition
Age | 26-43 |
Gender | Female, 50.3% |
Happy | 49.7% |
Calm | 49.7% |
Confused | 49.6% |
Disgusted | 49.6% |
Surprised | 49.6% |
Angry | 49.6% |
Sad | 49.7% |

AWS Rekognition
Age | 20-38 |
Gender | Male, 50.3% |
Surprised | 49.5% |
Disgusted | 49.5% |
Angry | 49.5% |
Calm | 49.5% |
Sad | 50.2% |
Happy | 49.6% |
Confused | 49.5% |

AWS Rekognition
Age | 20-38 |
Gender | Male, 50.3% |
Calm | 49.6% |
Angry | 49.7% |
Happy | 49.6% |
Sad | 49.8% |
Disgusted | 49.5% |
Confused | 49.7% |
Surprised | 49.6% |

AWS Rekognition
Age | 38-57 |
Gender | Female, 50.5% |
Disgusted | 49.6% |
Surprised | 49.5% |
Angry | 49.6% |
Happy | 49.6% |
Calm | 49.7% |
Sad | 49.8% |
Confused | 49.7% |

AWS Rekognition
Age | 19-36 |
Gender | Female, 50.2% |
Disgusted | 49.5% |
Surprised | 49.5% |
Angry | 49.5% |
Happy | 49.5% |
Calm | 49.6% |
Sad | 50.3% |
Confused | 49.5% |

AWS Rekognition
Age | 26-43 |
Gender | Female, 50.2% |
Happy | 49.6% |
Disgusted | 49.5% |
Surprised | 49.6% |
Angry | 49.8% |
Calm | 49.6% |
Sad | 49.8% |
Confused | 49.6% |

AWS Rekognition
Age | 4-9 |
Gender | Female, 50.2% |
Disgusted | 49.5% |
Sad | 50.2% |
Calm | 49.6% |
Surprised | 49.5% |
Confused | 49.5% |
Angry | 49.5% |
Happy | 49.6% |

AWS Rekognition
Age | 35-52 |
Gender | Male, 50% |
Sad | 49.6% |
Happy | 49.7% |
Calm | 49.8% |
Surprised | 49.6% |
Angry | 49.6% |
Disgusted | 49.6% |
Confused | 49.6% |

AWS Rekognition
Age | 26-43 |
Gender | Female, 50.4% |
Surprised | 49.5% |
Angry | 49.6% |
Happy | 49.6% |
Sad | 49.8% |
Calm | 49.8% |
Confused | 49.6% |
Disgusted | 49.7% |

AWS Rekognition
Age | 26-43 |
Gender | Female, 50.1% |
Angry | 49.9% |
Surprised | 49.5% |
Calm | 49.8% |
Sad | 49.7% |
Happy | 49.5% |
Disgusted | 49.5% |
Confused | 49.5% |

AWS Rekognition
Age | 26-43 |
Gender | Female, 50.1% |
Surprised | 49.5% |
Calm | 49.6% |
Happy | 49.6% |
Sad | 50% |
Angry | 49.6% |
Confused | 49.6% |
Disgusted | 49.8% |
Feature analysis
Amazon
Person

Person | 99.1% | |
|

Person | 98.8% | |
|
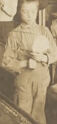
Person | 98.3% | |
|

Person | 98.2% | |
|

Person | 98.1% | |
|

Person | 98% | |
|

Person | 97.2% | |
|

Person | 97% | |
|

Person | 92.9% | |
|

Person | 84.1% | |
|

Person | 84.1% | |
|

Person | 84% | |
|

Person | 81.7% | |
|
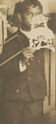
Person | 81.5% | |
|

Person | 77.4% | |
|

Person | 75.8% | |
|

Person | 75.7% | |
|

Person | 74.3% | |
|

Person | 74.3% | |
|

Person | 69.5% | |
|

Person | 69.3% | |
|

Person | 65.2% | |
|

Person | 61.8% | |
|

Person | 52.3% | |
|

Person | 48.5% | |
|

Person | 46.9% | |
|
Categories
Imagga
streetview architecture | 48.2% | |
| ||
paintings art | 19.6% | |
| ||
text visuals | 15.1% | |
| ||
interior objects | 13.6% | |
|
Captions
Microsoft
created on 2019-06-04
a vintage photo of a store | 55.6% | |
| ||
a vintage photo of a group of people in front of a store | 29% | |
| ||
a vintage photo of a group of people in a store | 28.9% | |
|
Text analysis
Amazon

York

Needs

Adaptation

Examples

New

Speeial

City

Education

the

Examples of the Adaptation of Education to Speeial City Needs

of

Public

Schools

to

New York City Public Schools 1161.716.7

Fret

Sawing

Vocotion

Vocotion Schoole

Brooklyn

Corpenter Shep Fret Sawing

Pub/

Schoole

Pub/ 5choof N932 Brooklyn

Corpenter

Shep

5choof

N932

1161.716.7

C7

New York City Public Schools
Examples of the Adaptation of Education to Special City Needs
31 heamrLTH
Vacation Schools
Fret Sawing
Carpenter Shep.
Public Sehool Ne3z Breoklyn

New

York

City

Schools

Examples

of

the

Adaptation

Education

31

Vacation

Fret

Public

Breoklyn

to

Special

Needs

heamrLTH

Sawing

Carpenter

Shep.

Sehool

Ne3z